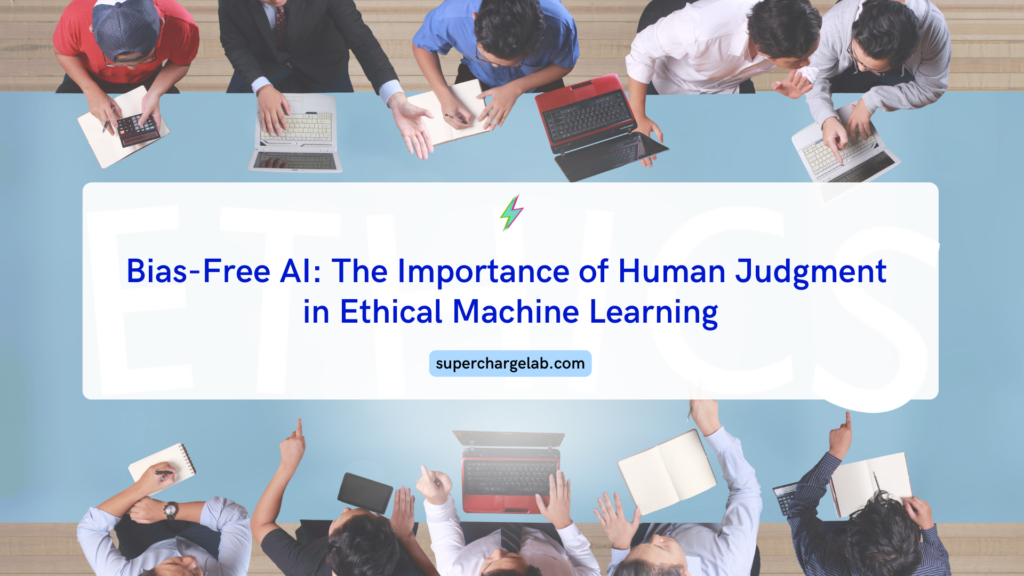
AI can be a powerful tool, but only if it’s fair. Shockingly, AI can be biased, reflecting the realities of bias in society. This highlights the need for human judgment in essential aspects of AI development, like ethical machine learning. To utilize the full potential of AI for good, we must prioritize fairness, ethics, and human judgment in its development and deployment. Let’s explore how human oversight can prevent bias and ensure ethical AI.
1. The Biased AI
AI algorithms learn from data, and if that data is biased, the resulting AI system will be biased as well. This phenomenon, known as algorithmic bias, can have serious consequences in various domains. For example, facial recognition systems have been shown to exhibit racial and gender biases, leading to misidentification and potential harm, especially for marginalized communities. In hiring, biased algorithms can discriminate against certain groups of candidates based on factors like gender, race, or age, perpetuating existing inequalities in the workplace. In criminal justice, biased algorithms can lead to unfair sentencing or parole decisions, disproportionately affecting individuals from certain backgrounds.
These examples are not isolated incidents; they highlight a systemic problem that requires urgent attention. The biases that exist in our society can easily seep into the data used to train AI algorithms, resulting in biased outputs that can have real-world consequences. This is why it’s crucial to address bias in AI to ensure that it serves all members of society equitably.
2. Human Judgment as a Safeguard
Human judgment plays a critical role in mitigating bias in AI. Diverse teams of developers, data scientists, and ethicists can identify and address potential biases during the development process. By bringing together diverse perspectives and experiences, these teams can anticipate and mitigate blind spots that might otherwise lead to biased algorithms. Ethical frameworks and guidelines can provide a structured approach to evaluating the potential impact of AI systems and ensuring that they adhere to principles of fairness, transparency, and accountability. For example, the “Fairness, Accountability, and Transparency in Machine Learning” (FAT/ML) principles provide a framework for researchers and practitioners to consider the ethical implications of their work and to prioritize fairness and inclusivity in their AI systems.
Human judgment is also essential in the deployment and monitoring of AI systems. Continuous oversight and evaluation are necessary to detect and correct any unintended biases that may emerge over time. Humans can also intervene when AI systems make decisions that are unethical or harmful, ensuring that AI remains a tool for good.
3. Building Trust in AI Systems
Transparency and explainability are essential for building trust in AI systems. Users need to understand how AI algorithms make decisions and what factors influence those decisions. This is particularly important in high-stakes domains like healthcare, finance, and criminal justice, where the consequences of biased or erroneous decisions can be severe. Explainable AI (XAI) techniques can help shed light on the inner workings of AI models, making them more interpretable and understandable to both experts and non-experts.
Involving stakeholders and diverse voices in the development and deployment of AI systems can also help build trust. Seeking input from affected communities and incorporating their feedback into the design process helps developers create AI systems that are more aligned with the needs and values of those who will be impacted by them. This participatory approach can help ensure that AI systems are not only technically sound but also socially responsible.
4. Regulations and Standards for Responsible AI
Governments and organizations worldwide are recognizing the need for regulations and standards to promote responsible AI development. The European Union’s General Data Protection Regulation (GDPR) includes provisions aimed at protecting individuals from discriminatory decision-making by automated systems. Other proposed regulations, like the Algorithmic Accountability Act in the U.S., seek to establish requirements for algorithmic impact assessments and bias audits, holding companies accountable for the potential harm caused by their AI systems.
Industry standards and best practices can also play a crucial role in mitigating AI bias. Organizations like the Institute of Electrical and Electronics Engineers (IEEE) have developed ethical standards for AI and autonomous systems, providing guidance on issues like fairness, transparency, and accountability. By adhering to these standards, companies can demonstrate their commitment to responsible AI development and contribute to a more trustworthy and ethical AI ecosystem.
5. A Human-Centered Approach to AI
Ultimately, the goal of AI development should be to create systems that benefit humanity as a whole. This means prioritizing human well-being, societal benefit, and ethical considerations throughout the entire AI lifecycle. It also means ensuring that AI systems are designed and deployed in ways that align with human values and principles, such as fairness, justice, and respect for human dignity. This human-centered approach to AI requires a shift in mindset from a purely technological focus to one that considers the broader social and ethical implications of AI. It involves asking questions like: How will this AI system impact people’s lives? What are the potential risks and benefits? How can we ensure that this technology is used for good and not for harm?
6. AI Works for Everyone
We can build a future where AI helps everyone if we focus on making it fair and unbiased. AI can solve big problems like climate change and disease, but only if we use it responsibly and think about how it affects people. Working together, we can make sure AI benefits everyone, no matter who they are or where they come from. https://calendly.com/superchargelab