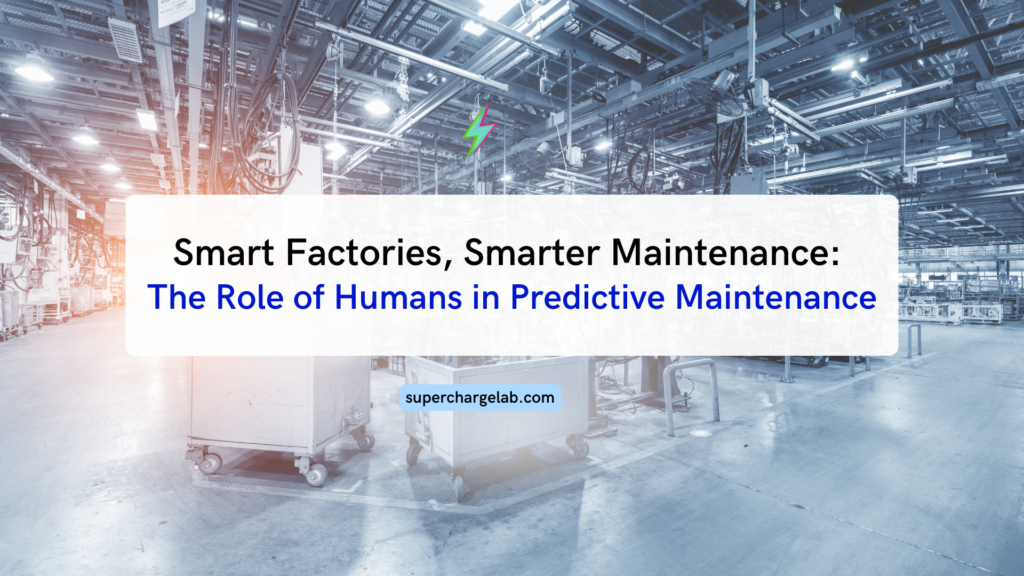
Unplanned downtime is the silent killer of productivity in manufacturing. But what if you could stop it before it starts? AI-powered predictive maintenance allows factories to predict and prevent equipment failures before they happen. Forget waiting for machines to break down, now its time discover how the technology is transforming the role of humans in industry 4.0.
1. The Rise of Smart Factories
Industry 4.0, also known as the Fourth Industrial Revolution, is characterized by the integration of digital technologies into manufacturing processes. Smart factories, equipped with sensors, robotics, and artificial intelligence (AI), are becoming increasingly common. These technologies offer numerous benefits, including increased productivity, improved quality control, reduced costs, and enhanced safety.
However, the rise of smart factories also presents new challenges, particularly in the area of maintenance. Traditional maintenance approaches, such as reactive maintenance (fixing things after they break) and time-based preventive maintenance (performing maintenance at scheduled intervals), are often inefficient and costly in this new landscape. They can lead to unplanned downtime, production delays, and unnecessary maintenance expenses. In a complex manufacturing environment, where even minor disruptions can have significant consequences, a more intelligent and proactive approach to maintenance is needed.
2. The Machine Whisperer
This is where AI-powered predictive maintenance comes into play. AI algorithms analyze data from sensors embedded in machines to detect anomalies, identify patterns, and predict potential failures before they occur. This allows maintenance teams to address issues proactively, minimizing downtime and maximizing equipment lifespan. Imagine a scenario where a sensor on a motor detects a slight increase in vibration. An AI algorithm, trained on historical data, recognizes this pattern as an early warning sign of a bearing failure. The maintenance team is alerted, and they can replace the bearing before it fails completely, preventing a costly breakdown and production loss.
Machine learning algorithms are the backbone of predictive maintenance systems. These algorithms can learn from historical data and continuously improve their accuracy over time, adapting to the unique characteristics of each machine and its operating environment. As more data is collected and analyzed, the algorithms become better at predicting failures, leading to even more efficient and effective maintenance practices.
However, implementing predictive maintenance in existing factories, especially those with legacy systems, can be challenging. Retrofitting old machines with sensors requires careful planning and execution to avoid disrupting production. In some cases, sensors may need to be installed strategically around the production line rather than directly on the machine itself. This requires expertise in both sensor technology and the specific production processes involved, highlighting the need for collaboration between engineers, technicians, and data scientists.
3. Humans,The Interpreters of AI Insights
While AI plays a crucial role in predictive maintenance, human expertise remains indispensable. AI algorithms can provide valuable insights, but it’s up to human technicians to interpret those insights, diagnose the root cause of potential problems, and determine the most appropriate course of action. A deep understanding of machine behavior requires a blend of human experience and domain expertise to grasp the subtle details involved.
For example, an AI algorithm might detect an unusual temperature increase in a machine component. A skilled technician can then investigate further, using their knowledge of the machine’s design, operating conditions, and maintenance history to determine whether the temperature increase is a normal fluctuation or a sign of an impending failure. This human-AI collaboration is essential for maximizing the benefits of predictive maintenance and ensuring the smooth operation of smart factories.
4. Preparing the Workforce for the Future
The rise of AI and automation in manufacturing necessitates a shift in the skills required of the workforce. While some tasks may be automated, new roles will emerge that require a different set of skills. Upskilling and reskilling programs are crucial to equip employees with the knowledge and expertise needed to work alongside AI systems.
Training in data analysis, problem-solving, critical thinking, and digital literacy will become increasingly important. Technicians will need to understand how AI algorithms work, how to interpret their output, and how to use this information to make informed maintenance decisions. This shift in skills will not only ensure that the workforce remains relevant in the age of Industry 4.0 but also create new opportunities for career advancement and job satisfaction.
5. The Future of Manufacturing is Collaborative
The future of manufacturing lies in a collaborative partnership between humans and AI. Smart factories, powered by AI-driven predictive maintenance and operated by skilled human technicians, have the potential to revolutionize the industry. Increased productivity, improved efficiency, reduced downtime, and enhanced product quality are just some of the benefits that can be realized through this collaboration.
Additionally, this collaboration can enhance job satisfaction for employees. AI can automate repetitive and routine tasks, allowing human workers to dedicate their time to more complex and fulfilling activities that utilize their unique skills and expertise. As technology continues to advance, the role of humans in manufacturing will continue to evolve. Embracing this change and investing in the workforce’s skills will ensure a bright and prosperous future for both manufacturers and their employees.
If your manufacturing or industrial business is looking to leverage the power of AI to improve efficiency, productivity, and safety, we invite you to contact us for a consultation. https://calendly.com/superchargelab